could any one support me by more explanation of error model, weighting schemes....I read boomer's tutorials and I understood it ....... but I found that I still did not cover all of the topic ,when I explored bonate's book .... if you have videos , tutorials , or even old topic on this fourm , please post here . ?
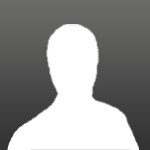
explaining of error models , weighting scheme
#2
Posted 31 July 2017 - 10:55 AM
Hi Mahmoud, can you maybe be more specific in your question. Is it how or why we use error models when fitting models to our data? For the former it is to improve the fit and therefore the precision of the parameter estimates obtained.
For the how this may be a useful place to start exploring the options in Phoenix models, there are other related threads too if you search on a term e.g. additve?
https://support.cert...residual-error/
Simon
#3
Posted 31 July 2017 - 03:11 PM
I mean there is a lot of models for variance and a lot of methods for estimation of it's parameters like that attached photo above ... Is there is any simple guide/ video that help in that ? should I understand that in this introductory level? or I will not use it now
#4
Posted 31 July 2017 - 03:27 PM
OK you mean how to choose the 'best' error model. Primarily ook at the residual error plots eg IWRES vs IPRED andIWRES vs IVAR
this shows you how different the observed and predicted concentrations are versus concentration and time respectively.
As a general rule you will find multiplicative tends to be better when your data spans seeveral orders of magnitude whereas an additive (also described as uniform) error might work better on e.g. PD data that tends to exhibit less range. It is a case by case situation, I suggest to copy you model and try different error models and see if thye improve your fit; with experience and practice you will find much easier.
Simon
Also tagged with one or more of these keywords: error, error model, weighting, weighting scheme, modeling of variance, explansion of variance
Certara Forums →
Phoenix WNL basics →
PE and 90%CI Not Estimable (Invalid Model)Started by BEQool , 12 Mar 2024 ![]() |
|
![]()
|
||
Certara Forums →
Modelling and Simulation →
Select residual error model and VPC plot with negative valueStarted by joybaker , 19 Nov 2022 ![]() |
|
![]()
|
||
Certara Forums →
Modelling and Simulation →
Error in setting covariatesStarted by vetpharma , 01 Jul 2022 ![]() |
|
![]()
|
||
Certara Forums →
Phoenix WNL basics →
Data wizard error: Bad conversion from DBNULL to doubleStarted by Zhengguo , 30 Jul 2019 ![]() |
|
![]()
|
||
Certara Forums →
Phoenix WNL basics →
Failed to create table. Access to the path ... is deniedStarted by rosy_mtz , 21 Nov 2018 ![]() |
|
![]()
|
2 user(s) are reading this topic
0 members, 2 guests, 0 anonymous users